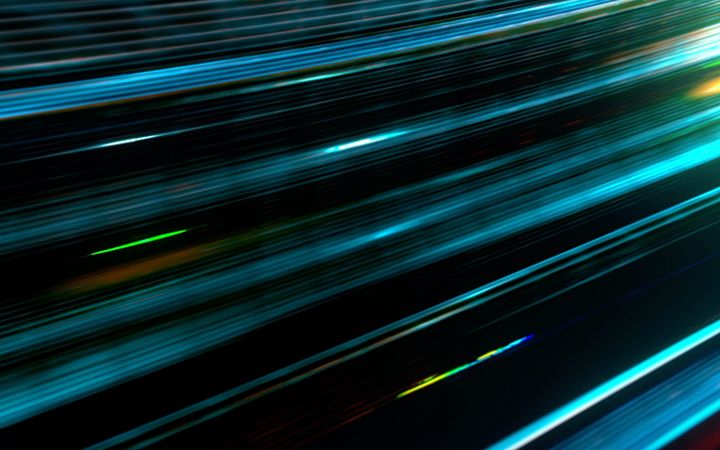
Health research is time-consuming and expensive. Can we do better without compromising accuracy?
From climate change to opioid addiction to the COVID-19 pandemic, we face serious public health crises that put our research and data management experts to the test. When it comes to scientific evidence, systematic literature reviews—painstaking assessments of all the literature ever produced on a given subject—are often regarded as the gold standard.
But health research is time-consuming and expensive. And its comprehensiveness comes at a high price in terms of time and money. It involves sifting through enormous volumes of literature—sometimes hundreds of thousands of scientific abstracts—stored in academic databases. Researchers use broad keywords to query these databases and capture as many results as possible, but the task of wading through the research falls to subject matter experts in what turns out to be a manual, time-intensive, and expensive process.
Start by understanding the research context
Before we can prescribe an IT solution, we need to understand the research question our clients are trying to answer, and what purpose the research will serve. For example, an agency may need to make decisions about a chemical. Will it affect human health? What are the potential impacts of exposure? Some chemicals are very data rich—they’ve been around for a long time and have been well studied, so there is a large volume of information to comb through. And other chemicals are emerging contaminants. Not much is known about them, so the data set is much sparser.
Two different chemicals. Two very different data sets. The size of the data set will inform the approach we take to processing it, as will the goal of your research. If you’re trying to put a chemical forward for regulations, you will need to have full confidence in your research—no faults whatsoever. Research of this nature takes more time. But if you’re conducting a prioritization screening exercise, you will favor speed over comprehensiveness, and the IT approach we recommend will be different as a result.
It’s essential to have an anchor in domain expertise when advising public health agencies on IT modernization initiatives. Artificial intelligence and machine learning tools can help researchers in both examples above, but the application of these technologies will vary based upon the data volume, speed, and accuracy needs of the research context.
Data retrieval vs. data extraction
The health research community has become more comfortable with automating data retrieval in recent years, but data extraction is a different story.
Consider first the data retrieval process. In a systematic review of health research, data retrieval involves writing a query for a database that throws out a net and pulls in 95% of the articles that have anything to do with what you’re looking for.
The biggest risk with data retrieval is that you'll miss some of the relevant articles or data. Your data retrieval net might pull in irrelevant data, but you can weed that out later. The important thing is to retrieve as many of the relevant articles (or data) as possible.
Data extraction is not about casting a net. Instead it’s about mining for the important things. You’re going into each of those articles you retrieved to extract the relevant information. There are two risks: you might miss extracting data that’s actually important to answer your research question or you might extract the wrong data that doesn’t help answer the question. Using machine learning for data extraction is a higher-risk proposition because, in the end, this extracted data is what you’ll use to answer the research question and draw conclusions and you may lose context or miss an important finding.
The reliability of machine learning
There is room for machine learning in data extraction, but health researchers are understandably apprehensive. Some fear machine learning processes will be inaccurate, will somehow not get the right data out, or will change the meaning of that data.Regulators require that machine learning omits no more than 5% of relevant articles or publications. When relevant data are omitted, imprecise conclusions may be drawn, which may impact confidence in conclusions and ultimately lead to regulations based on partial evidence. However, the biggest concern is whether these omitted articles are inherently different in conclusion, despite similar original hypotheses, such that the omission leads to a systematic bias in the conclusions drawn.
Supervised clustering—based on the theory of semi-supervised learning—can classify documents and generate accurate and unbiased estimates of model accuracy with a minimal training dataset.
In a research paper, we used two previously classified datasets related to chemical toxicity to demonstrate the accuracy and lack of bias in model performance metrics generated by supervised clustering. Simulations showed that the accuracy and manual work saved using our supervised clustering method is comparable to the performance of more expensive supervised machine learning-based methods.
While this and other methods can potentially reduce training dataset size compared to random sampling, they potentially suffer from biased accuracy predictions that negate their potential benefits. In other words, when an active machine learning algorithm tells a user that the user can stop training it and makes predictions on the fraction of relevant documents contained in the discarded documents, it may be omitting more relevant articles than it declares. This poses serious problems in the regulatory context.
Can a machine learning solution help your agency meet its mission?
The cost savings and efficiencies offered by machine learning make it inevitable that this technology will be critical to health research. Already, it has proven to be a powerful tool for helping our own clients make decisions more quickly, and with more data-backed authority.In the regulatory environment, however, issues around legal and scientific defensibility still present some potential barriers to the adoption of machine learning. The future of machine learning in health research will continue to require careful interaction among subject matter experts, technologists, and computer programmers.
Not to mention plenty of very human quality control.