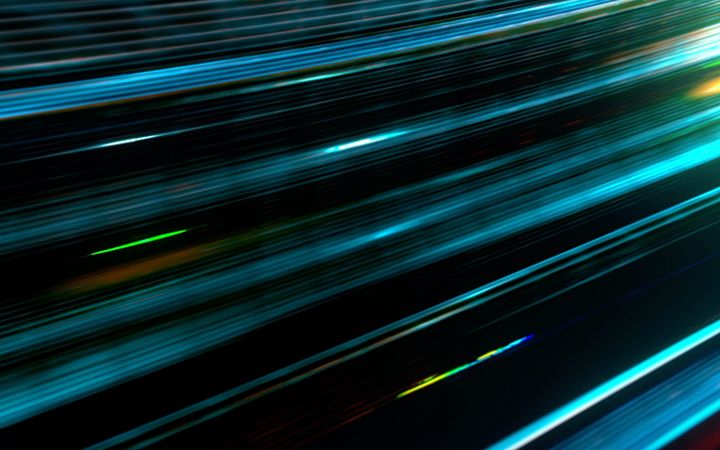
What data science can do about our mental health state of emergency
The pandemic continues to have a deep and wide-reaching global impact. Grappling with the virus and mounting an unprecedented worldwide response has shone a light on public health—and brought into high relief its challenges, capabilities, and limitations. As the pandemic continues to recede, something worrisome is being revealed: there is a global mental health crisis upon us for which we are just as unprepared.
Inadequate testing, measurement, treatment, surveillance, difficult access to care, unreliable information; the similarities are many. There are key differences, not least of which are the private, often invisible, and poorly measured experiences of mental illness. We have also underinvested in our capabilities to respond to a mental health state of emergency compared to investments in physical, biological illness. Large swaths of the country have little or no access to the appropriate care. Those that do have access have to navigate deep ruptures of trust, stigma, and variable provider competence.
There have been widespread failures to evolve our data capabilities beyond describing the etiology and prevalence of mental illness, to advancing our ability to do something about it. Researchers, practitioners, and policymakers must make every effort to benefit from the modern capabilities that data science—developing methods of recording, storing, and analyzing data to effectively extract useful information—provides to blunt the impact of this pandemic and improve our ability to predict, prevent, and cure mental illness.
Mental health in the U.S.
The numbers are alarming. In the U.S. alone, as many as one in every five people experience mental illness and fewer than half receive treatment for it. That is more than 25 million people who are not receiving care. Incredibly, an average of 11 years elapse between the first symptoms and starting treatment for those who are lucky enough to have care. Suicides continue to be on the rise, with stark figures revealing that it is the second leading cause of death for the 10–34-year-old population.
This younger segment of the population is experiencing an increase in mental illness, with one in every five or six children from 6-17 years old having a treatable mental health disorder. Diagnoses in this age range in the U.S. increased 30% between 2011 and 2017. Data covering the period from 2012 to 2016 show a 55% increase in mental health emergency department visits from this age group.
More recent data from 2020 show a further acceleration of emergency department visits—by 24% for children from 5-11 years old, and 31% for those 12-17 years old—compared to 2019. These data that relate to young people are particularly disturbing because research indicates that 50% of all lifetime mental illness begins by age 14 and 75% by age 24. As those with mental illness enter adulthood, individuals with the greatest disability will contribute almost 14% of all years of life lost to disability and premature death.
Data science challenges
Mental health research and practice is a complex ecosystem with endless challenges. To help distill these and focus on the most vital and pressing priorities, leaders in mental health assembled a compendium of Grand Challenges. This included the goal of identifying root causes, risk and protective factors including biomarkers, gene-environment interactions, and social context.
Also included was the advancement of prevention and implementation of early interventions including developing evidence-based primary prevention, and assessment of neuroprotective agents to reduce vulnerability to disorders in adolescence. A third goal included improving treatments and facilitating access to care by integrating mental health into primary care and expanding access to treatments and decision support for non-specialists. These challenges are at their heart data science challenges.
Data science advances—tools, technology, techniques, and frameworks—offer promising accelerators for transforming mental health and create opportunities for breakthroughs in tackling this pandemic. The full value of data science has not been realized with respect to mental illness, despite data science being a formidable driver of discovery and advancing our ability to predict, prevent, and treat a very wide range of physical illnesses. We require an energetic commitment to data science and optimized behavioral research to advance a data-driven understanding of mental illness and sharpen our tools for early and effective intervention.
Addressing data poverty
The mental health data ecosystem is poor, especially when compared to its biomedical neighbors, which has delayed a broad adoption of routine data science practices. Publicly available data regarding mental health is limited by modest scale and depth, variable quality, and heterogeneous standards and formats. Mental health practitioners and researchers looking for data are often forced to rely on trial and error, and haphazard discovery, delaying research breakthroughs and effective interventions.
Data that does exist often represents only those in treatment or trials, but not the total population of people grappling with mental illness. Data rarely aligns with the FAIR (findability, accessibility, interoperability, and reusability) data principles, and is not in a ready state for advanced techniques like artificial intelligence and machine learning.
Mental health professionals can address data poverty by acquiring the data science skills to work with data; contributing data, curating data, prioritizing data, and integrating data into their practice and research; creating culture change in how we view data, and evaluate our work in relation to data; and sharing openly important and reliable data sets (e.g., collaborative biobanks).
Democratizing access and facilitating collaboration
Data silos and effort islands will restrain our progress in addressing mental health. As a community, we need to facilitate cooperation on a global scale in the conduct of research and enable open, democratic access to data, tools, technology, expertise, and the opportunity to build greater cooperation. The critical path item for collaboration is having a reliable and accessible place where data lives. In biomedical research adjacencies, this has been accomplished with data commons models.
Mental health data commons can support an open, standardized, credible, elastic, and collaborative home for robust, reliable, FAIR, and interoperable mental health data. Furthermore, a commons model provides an infrastructure that gathers, harmonizes, and creates access to relevant data sets and connects these with tools to allow users to share, integrate, analyze, and visualize mental health research data and discoveries. This colocation of data with tools can assist researchers of a wide range of backgrounds to make queries, hunch associations, and create and test hypotheses.
Making sense of mental illness' complexity
Data science is our ally in disentangling the complex etiological paths, interrelation of physical and mental disease comorbidity (the simultaneous presence of two or more illnesses, physical or mental), and its setting within social and cultural milieux. Classical techniques alone are not powerful enough to identify and understand many of these complex processes.
The challenge is broadly acknowledged: people struggling with mental illness are at much greater risk of developing and suffering from physical illness, and report higher rates of respiratory disease, heart disease, diabetes, and other illnesses. Some data are even beginning to indicate a relationship of mental illness to cancer prevalence. Data science can help better understand, prevent, and treat patients who are presenting with comorbid illnesses.
Data science can also better describe and analyze the complex system of social, cultural, and economic factors within which deterioration and active mental illness take root. Factors that comprise the social determinants of health (SDOH) are acknowledged to have a substantial impact on mental and physical health outcomes, highlighted by the very high rates of mental illness and suicides reported in different populations. Using data and analytics to understand the way that these factors trigger and contribute to illness is key to bringing change through enabling access to care and resources, mental health education, destigmatizing mental health, and addressing policy and structural contributors.
Using tools and techniques to pivot from reactive to preventive
Artificial intelligence, machine learning, natural language processing, semantic analysis, and predictive analytics are all tools with legitimate and important utility to mental health research and practice challenges. These data science capabilities can help create meaningful associations between disparate data, advance prevention, early intervention, and reduction of early life vulnerability. These capabilities are fully up to the task of tackling the deleterious effect of social media and using its data to predict and interrupt mental health risks—including suicide. This includes predictive models that make possible early intervention to disrupt disintegration into mental illness with an easy-to-use, data-driven (and generating) screening system so that protective countermeasures can be taken.
Data-science-enabled screening could be used at the primary care level and be implemented and interpreted by non-mental-health-specialist practitioners. At the population, public health level, such models also enable the massing of resources in more precise and targeted ways to educate and prepare larger groups of people to treat and prevent mental illness.
Using data science to expand access to care
Quite apart from there being limited care available, the public does not have sufficient awareness and understanding of the variety of mental illness treatment modalities that do exist. The Kaiser Family Foundation study has found that most states have met less than 50% of mental health care needs among their populations. The study identified a shortage of providers and racial and ethnic disparities as major causes.
Data science can facilitate the connection of people struggling with mental illness with the most effective treatment so that they remain engaged and maximally benefit from treatment encounters. This will include the expansion of access to mental health telehealth, which serves a great number of communities but in particular rural ones that have provider shortages.
Enabling more representative research
Data science can help connect the public with opportunities to engage with research programs and reduce barriers to accessing clinical trials. This serves the critical objective of enabling research and data that is more representative, accessible, and timely. Psychiatric and mental health clinical trial participation suffers from some of the sampling inadequacies of its biomedical neighbors, including a reliable skew away from certain groups participating. Many variables contribute to this problem including unaddressed culture and language factors, barriers to accessing care and participating in research, and the enduring ruptured trust created by programs like the Tuskegee study.
Data science can be used to create more representative research, and support better enrollment and participation in clinical research trials and their results. Technology exists to enable virtual study participation, e-consent, remote data collection, use of apps and wearables, coordination of many disparate electronic workflows and data streams, and collection of real-world data.
Making space for data science
We are faced with a pandemic of mental illness, which should force us to adjust our approach to both research and practice, and to improve prediction, prevention, and cure. Data science—as a set of capabilities comprised of tools, methods, algorithms, and systems to identify relationships and create insights in complex, very large-scale data—can help us address this pandemic emergency. Historically, data science has not enjoyed the same traction in the mental health domain as in other biomedical adjacencies. This has to do in large part with data poverty and a non-native skillset to take advantage of data science capabilities.
Data science is an accelerator that does not replace traditional mental health practices but enhances them, and helps make sense of complexity. These techniques can create timely insights and predictions that favor action and prevention of illness. The use of data science and associated techniques and technologies can also bring more people together to collaborate around mental health’s toughest challenges. Critically, data science can help more effectively connect people from a broad range of backgrounds living with mental illness with the right treatment and opportunities to participate in clinical trials.
Much must be done to address this pandemic of mental illness, and it is quite clear that a status quo approach is not sufficient to create the sorts of breakthroughs that are needed. Across the sector, we need to focus on training the workforce to meet our mental health needs more adequately. This includes changing the culture by providing clinical and research mental health professionals with the skills and opportunity to apply data science techniques to their work and determining new opportunities for integrating its capabilities.