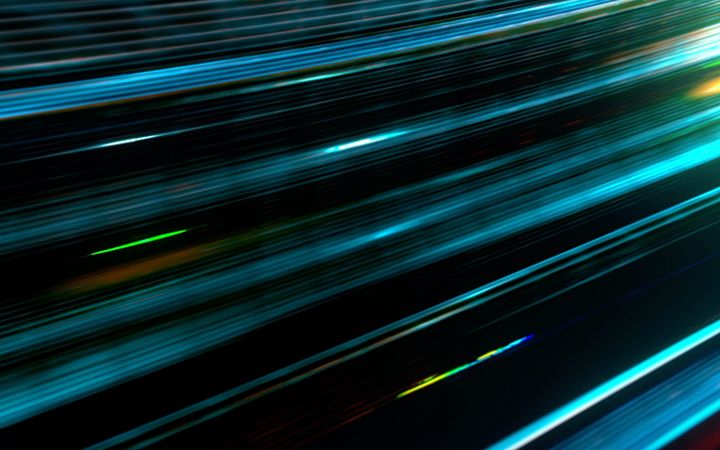
Strengthening health preparedness with better data forecasting
Accurate public health forecasting requires the supply of consistent, high-quality data. Yet in the United States, there is no single clear pipeline to provide such data among local, state, and national levels. Those disconnects can severely hamper a collective and appropriate response to public health threats.
It’s also crucial to ensure that the data feeding public health forecasts account for the ways race, ethnicity, and other factors can affect health outcomes. Recognizing this challenge, the Robert Wood Johnson Foundation created a National Commission to Transform Public Health Data Systems. One of the commission’s three overarching recommendations was to “ensure that public health measurement captures and addresses structural racism and other inequalities.”
Gaps in the data-sharing pipeline, in addition to a lack of access to consistent, representative health data, have consequences. These shortfalls can contribute to inaccurate forecasts that are not useful to—and may even mislead—those charged with making critical public health decisions. An inaccurate forecast will not be useful to the public and may erode the public’s trust in institutions they expect to help them. Therefore, streamlining and improving the collection and sharing of public health data is of paramount importance if we wish to respond effectively to future threats, pandemic or otherwise.
Building an accurate and fair forecast
To create an accurate forecast that will prepare localities and health systems for forthcoming threats, you need to do three things:
- Understand the underlying science for novel viruses and health threats, including the most at-risk populations and the factors that might influence its impact.
- Collect the most comprehensive and representative data, which systematically accounts for factors such as race, ethnicity, and disability status.
- Build an accurate and fair algorithm, accounting for the nature of the event, the populations who are most vulnerable to it, any shortcomings in the underlying data and the possible environmental, socio-economic, and behavioral variables that might affect the forecast outcome.
As the CDC and other government entities learned painfully during the COVID-19 pandemic, collecting the best data is not easy. These organizations, as well as hospitals and health care providers, historically have faced many challenges in their efforts to collect an appropriate percentage of reports that accurately list race and ethnicity. For example, a significant amount of infectious disease reporting comes from laboratories, yet lab specimens typically have limited patient-related data attached to them such as a name, birth date, or gender. Even in aggregate, the CDC and other public health organizations need more comprehensive information in order to fully understand who is most affected and at greatest risk.
What’s more, when data do include race and ethnicity, the degree of granularity may not be sufficient to accurately identify a population’s risk. For example, the race option “Black” encompasses several discrete communities, including African Americans whose families have been in the United States for hundreds of years as well as Africans who recently immigrated to the country. It includes people from Caribbean countries whose race is listed as “Black” but who ethnically are from the Dominican Republic, Haiti, or Barbados. The impact of a disease or event among these different sub-populations may be hidden if they are lumped together.
Finally, national data often do not accurately represent extremely small populations. This is a chronic issue for Native American/Alaska Native people whose numbers account for a small percentage of the national population. Data collected for these groups may not be adequate for models to find statistically significant trends, making it difficult to detect health signals among these populations.
When variations like this aren’t reflected in data, there can be significant consequences. For example, health care providers aren’t routinely asked questions about whether patients have disabilities on disease-reporting forms. During the COVID pandemic, this was a problem because it made it more difficult to document the higher mortality rates for these populations: This delayed the development of specific guidance for that population.
A multidisciplinary approach to data modernization
Improving the collection and sharing of data requires a thoughtful, multidisciplinary approach. At ICF, we house several areas of expertise, including epidemiologists, biostatisticians, survey design and implementation specialists, experts in probabilistic and non-probabilistic sampling methods, technologists, communications professionals, and government agency veterans. We bring these minds together, focus group-style, to address client challenges from multiple angles. These capabilities allow us to design instruments and technologies that can help agencies provide comprehensive, unbiased insight that can help yield accurate, actionable public health forecasts.
Take, for example, the Behavioral Risk Factor Surveillance System (BRFSS), a three-decade partnership between ICF and more than half of U.S. states and territories. Governments use data from this annual survey of more than 400,000 people to help make public health decisions to benefit their residents. Through this partnership, ICF has merged deep expertise in data collection and sampling protocols with a state-level understanding of population, region, and health priorities. The BRFSS is a great example of how agencies and providers can meaningfully collect complicated data in small subpopulations. Its flexibility increases the likelihood that data produced are relevant to the complex planning needs of each locality that participates.
Another example is BioSense, a hospital-based program designed to identify disease outbreaks based on the analysis of medical records. The CDC and the Division of Health Informatics and Surveillance partnered with ICF to upgrade the technology of the BioSense platform and improve the reach and quality of its surveillance data. Bringing together experts in public health, digital transformation, information management, data management, and analytics support, ICF helped the agencies overcome challenges related to data sharing and ownership, as well as data aggregation and suppression. The result was an increase in the ability of local, state, and national health officials to monitor and quickly detect priority public health concerns on a broad scale.
Better data, better forecasts, and better health outcomes
In both preceding cases, ICF’s multidisciplinary approach and its attention to the meaningful engagements of federal, state, and local agencies and the affected populations helped health organizations collect higher quality data and create pathways for that data to be shared across regions, states, and the country. These efforts have increased our partners’ capacity to create accurate forecasts that can help leaders at all levels make wise, timely, and equitable public health decisions. This work is essential to protecting the health of the public from coast to coast, whether it’s preparing the nation’s health systems for a routine flu season or responding to an emerging pandemic.