Our advanced health technology and data solutions are informed by deep expertise in epidemiology, genomics and proteomics, and more—all applied to meet organizational requirements, deliver effective programs, and implement data-driven solutions.
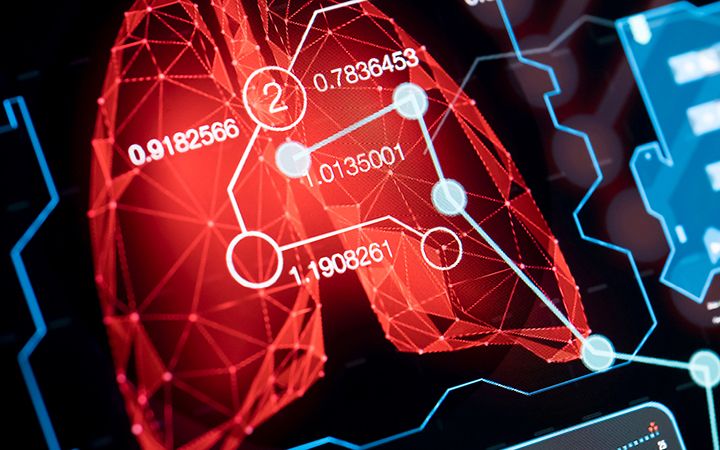
Translate data into better health outcomes
Our experts work with your enterprise data—modernizing your infrastructure and developing data strategies that align to outcomes—to help you apply it in program, research, and clinical settings. We unify different data sources to unlock deeper insights, improve treatments, and boost survival rates.
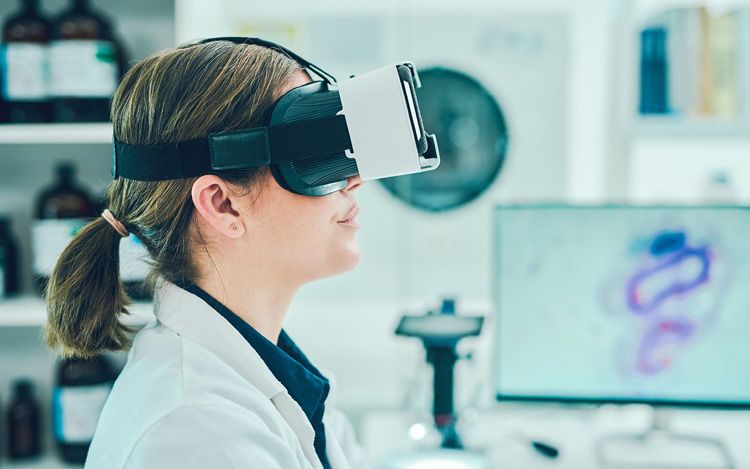
Unlock health insights with bioinformatics
Our bioinformatics solutions turn complex, large-scale health data sets into actionable insights. We integrate large data pipelines with advanced analytics and visualization tools to support scientific discovery, improve patient care, and advance health outcomes.
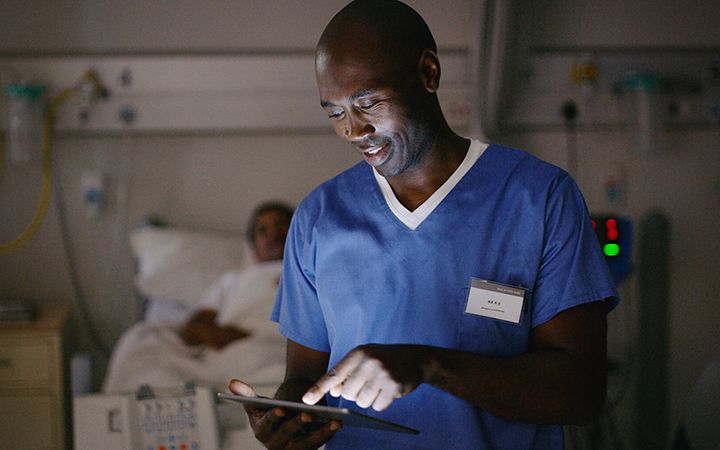
Seamless health data integration
We develop standards for health data interoperability so information can flow easily and securely between systems. We work with standards development organizations to integrate health data pipelines so you can deepen your data’s reach.
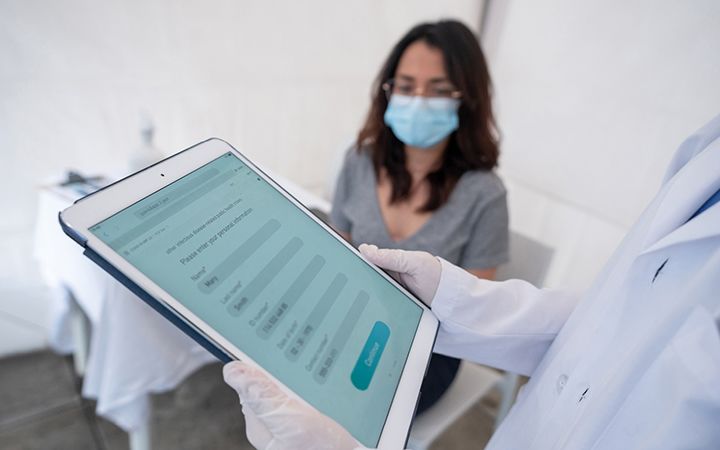
Drive digital modernization
As federal health agencies shift from legacy IT to agile, secure, and cost-effective systems, our software development, systems integration, and low-code/no-code solutions enable seamless health information exchange.
Other related services
Health IT
- Software development
- Data interoperability and systems integration
- Digital transformation
- High performance computing
- Scientific research platforms
- Health surveillance systems
- Policy and standards development
Data management
- Data and analytics strategy and governance
- Artificial Intelligence
- Cloud and open source
- Predictive analytics
- Fraud, waste, and abuse detection and prevention
- Lab data exchange solutions
- Portal development
- Quality assurance
Life science and bioinformatics
- Data science and analytics
- Microarray analysis
- Genomic sequencing analysis
- Visualization tools
- Clinical genomics
Our work
Our insights
Our technology partners
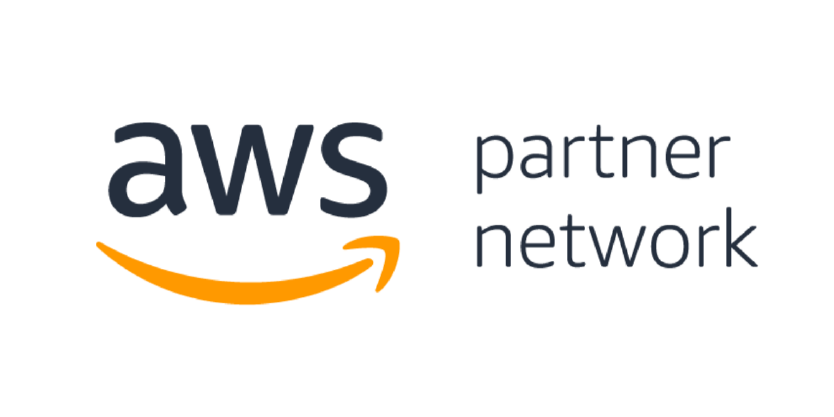
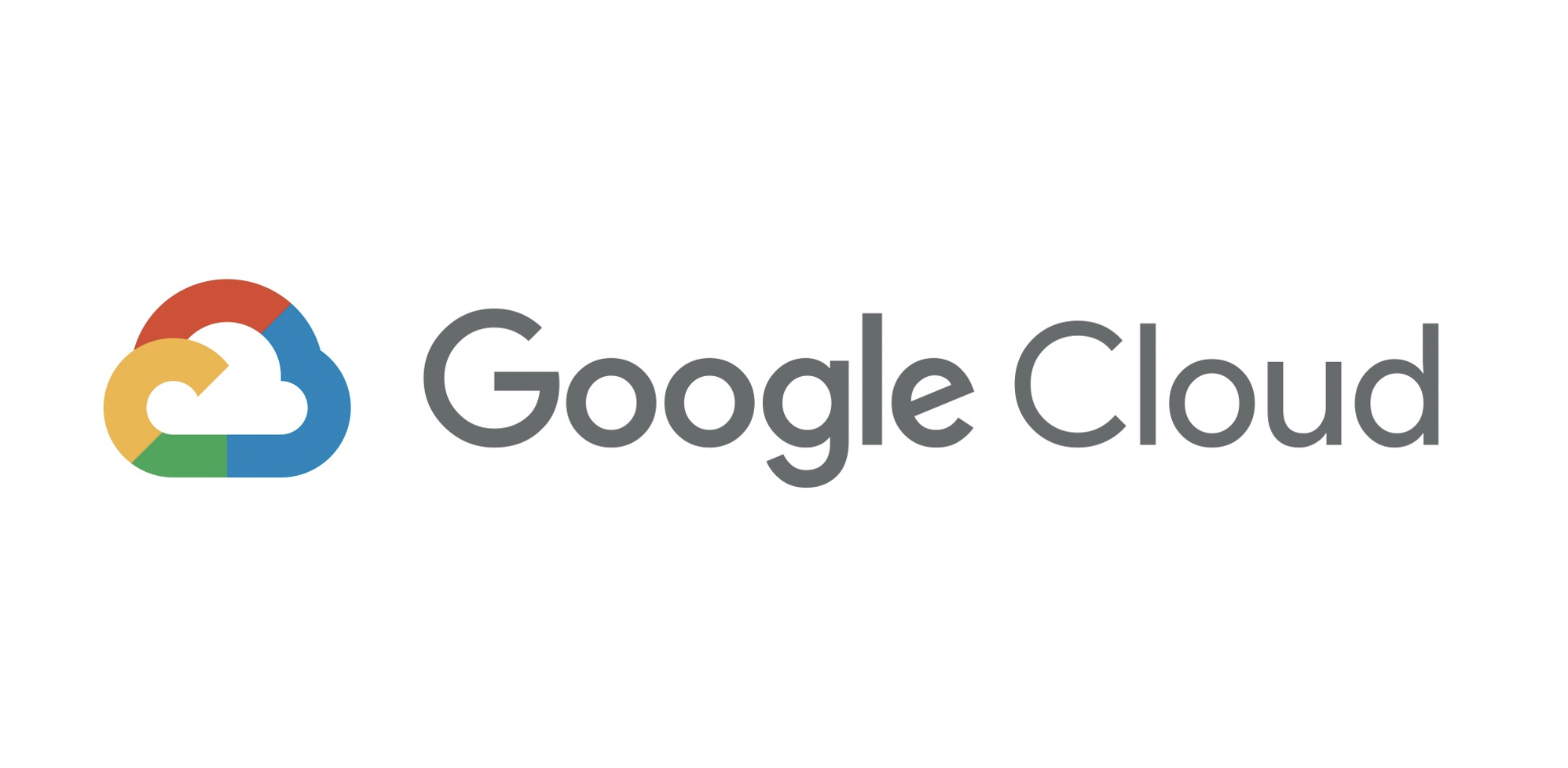
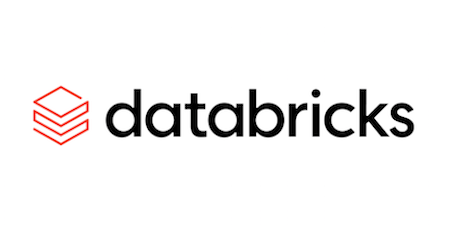
Related industries, solutions, and services