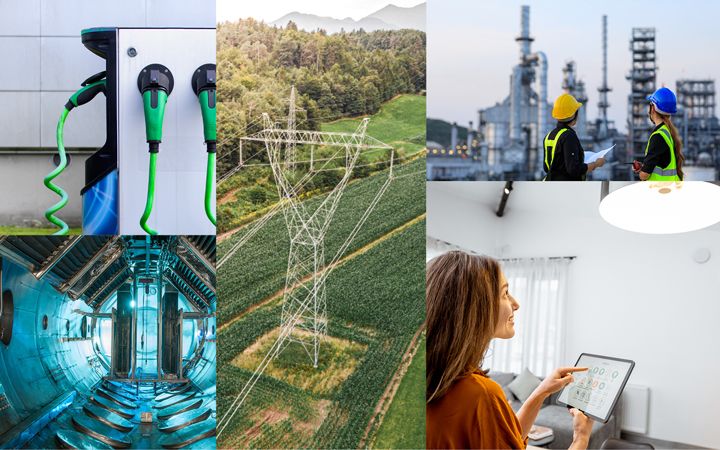
AI in action: Using digital twins to help utilities manage demand growth
With the growing role of artificial intelligence (AI) in our work environments and everyday lives, there’s no shortage of opinions or predictions of what AI can accomplish. But what are we learning when we cut through the hype and start applying AI in the real world?
In this article, we’ll turn our attention to the energy sector and a promising application of AI that allows utilities to be more efficient and targeted in their program efforts. Is that how AI actually works? Let’s find out.
The Situation: |
How can utilities use AI to plan better, faster programs to manage energy demand? |
The Subject: |
Digital twins |
The Experts: |
Haider Khan, vice president of energy analytics; and Gregory Donworth, senior manager of data science |
Q: Are utility program leaders interested in AI?
Haider Khan: The utility sector is experiencing rapid electricity demand growth right now. Program leaders must plan and implement energy efficiency and load management programs that meet their energy reduction goals at a time of rising energy use. They also have a number of other goals they need to achieve simultaneously, like reducing energy costs for low-income communities.
While they’re very interested in the possibilities of AI in automating these processes, the technology landscape is crowded, noisy, and rapidly changing. Program leaders are always looking for help in determining which technologies—or combination of technologies—will provide value now and in the future.
Q: It seems like the term ‘digital twin’ is popping up all over the place. What is it?
Greg Donworth: Digital twin is definitely an up-and-coming keyword that everybody's interested in hearing more about these days. I think it’s one of the more exciting ways that we’re using data science to solve real-world challenges.
Most people are familiar with the concept of building a scale model of an actual building. Then physics-based modeling came along and allowed us to create a simulated model, or digital twin, where we could apply mathematical equations that represent the building’s behavior when subject to various conditions—think weather, in-home appliances, HVAC systems, etc.
Now we’re in the realm of cloud computing. In the same amount of time that it used to take to analyze one building (e.g., the heat transfer that's happening in the air circulating inside the building), we can now create digital twins of all the buildings in a utility territory.
Q: Ok, so how can utilities actually use digital twins?
Haider Khan: At a very granular level, they can use digital twins to target specific homes or businesses to find out if the building has the right amount of insulation for the weather conditions and forecast how much insulation would be cost effective for them. They can also zoom out and look at the current and forecasted impact of different customer programs (including solar, storage, and energy efficiency technologies) in a specific region or their entire jurisdiction.
Where it gets really exciting for utilities is in identifying and forecasting remedies for congested locations on the electric grid, anywhere from one minute to 30 years into the future. With parallel computing, they have access to a lot of predictive power. Using digital twins, utilities can query their grid with questions like, “What are the top 10 locations in my service territory with the highest demand for electricity and what available resources can shift or shed that demand?” This works at the substation level and the building level.
Q: As of today, who’s using digital twins? And where does it make sense to go next?
Haider Khan: The use of digital twin technology is expanding across numerous industries. Specifically in the energy sector, digital twins are being applied for basic supply planning around Distributed Energy Resources (DERs) like solar panels and EV charging stations within the grid.
We work with utilities that are now seeing that they can use digital twins in many different ways. All the way from high-level planning—power plant planning, transmission planning, and distribution planning—down to how to increase energy efficiency through better design and faster implementation.
Key insight: We now have the computational power to make digital twins of everyone and everything.
Q: What technologies do organizations need to have in place for a well-functioning digital twin?
Greg Donworth:There are three core capabilities necessary to establish a well-functioning digital twin analysis. First, you must have the foundational knowledge of the system that the digital twin will imitate. For utilities looking to create digital twins of the buildings on their grid, this includes a deep understanding of the physics of heat transfer, fluid dynamics, and thermodynamics.
Second, you need access to vast amounts of cleaned and organized data that can validate and improve performance. Utility forecast models use machine learning to build algorithms based on past energy consumption, and then adapt them for future circumstances based on normalized load growth due to trends like EV adoption; it’s a lot of labeling of data to train the data.
Third, cloud technology is necessary to create and run digital twins at scale. At ICF, our most recent model across an entire utility’s jurisdiction required creating and running 2.3 million digital twins—one for each of their customers—to forecast the performance of various technologies over a period of 30 years. This requires massive computing capabilities only available through the cloud.
WHAT WE LEARNED: With AI, utilities can now create a digital twin of the most congested areas of the grid to predict the incentives and programs that customers will adopt. This could work for flexibility, DERs like rooftop solar, energy efficiency rebates, and more. AI can help the utility figure out the best solutions for managing their electric system by leveraging advanced metering infrastructure (AMI) data through digital twins of buildings and the distribution system.